
Can Artificial Intelligence be the Answer to Every Analytics Problem?

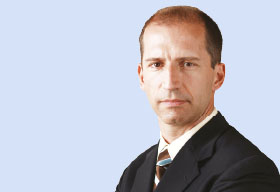
Although there is no doubt that AI can be a powerful tool, we see certain risks and limitations. The three risk factors of AIare:(1) black-box nature, (2)false positives,and (3)80 percent quality issue.
Being a black box is an inherent property of most AI methodologies due to the nature of the technologies and algorithms used. While blackbox algorithms have been shown to produce useful results, the work only if every thing is used correctly and the risks and limitations are fully understood. If an error occurs due to an incorrect application, the audit trail may fail. Unfortunately, no body will be able to reconstruct what happened in the black box. To avoid such errors, we would need independent human controls to check what the AI is doing as well as fully understand the risks involved.
False positives are the second issue. "Intelligent search engines" generate millions of search results, but considering the volume of result produced, it can be a real challenge to identify the relevant ones. Irrelevant results are called false positives, while relevant results that are missing are called false negatives. Every body wants to minimize the false positives and the false negatives – this creates an inherent trade-off. An intelligent search engine should not only capture all the relevant results but
also remove the unwanted ones. However, if the cost of making a mistake is too high and you need certainty that there are neither false positives, nor false negatives, human minds are still essential, because it involves true judgment based on multiple factors that are not even contained in the data.
The 80 percent quality issue relates to cases where the quality of the product needs to be very high – 100 percent or close to it. Even the slightest doubt that the result may be incorrect or incomplete could be too big a risk. The only way to gain 100 percent certainty is by way of manual rework. The real issue here is that you need to redo the work for 100percent of all the records, not just for the incorrect 20percent. This effectively renders the AI obsolete.
However, in certain steps of the analytics value chain AI performs more efficiently and quickly than even the smartest data scientists. AI is particularly helpful when large amounts of data or information are involved. On the other hand, the human mind is still crucial when it comes to the ability to spot and react to new patterns (as opposed to known patterns);understand the broader business context; differentiate between correlations and root-cause relationships; and tell machines what to do. These traits are key ingredients for creating insights or knowledge and will continue to be dominated by humans, at least in the foreseeable future.
How can we make this mind+machine collaboration work profitably for many, vastly different and dynamic analytics applications? The answer is by eliminating clutter. End users do not have the time to review lengthy data sets or reports filled with information. They want concise insights to inform their decisions. Useful AI-based algorithms can plough through large data sets, remove the unnecessary clutter, and provide results by relevance. This is where the machine works best and accomplishes the task that humans would not be able to do at all (or only very slowly). A human analyst reviews the results offered by the machine and make a final decision on whether they are relevant or not. This judgment is end-user specific and adds context to the result – something that is still out of reach for even the most intelligent algorithms.
If each decision the human analyst makes gets incorporated into the machine through machine-learning techniques, the algorithm may get better at deciding the level of relevance. Only the results that have been validated by the analysts will then be passed on to the end user. Helpful as it may be, AI currently contributes to only a part of the value chain. The vast majority of AI applications still need a mind element to create truly useful insight. On top of this, most analytics solutions include many other machine elements, such as tools for workflow, productivity, dissemination, and knowledge management. AI is usually just the icing on the cake. Rather than being the answer to every analytics question, AI can be a very powerful tool when used appropriately.
Useful AI-based algorithms can plough through large data sets, remove the unnecessary clutter, and provide results by relevance
The 80 percent quality issue relates to cases where the quality of the product needs to be very high – 100 percent or close to it. Even the slightest doubt that the result may be incorrect or incomplete could be too big a risk. The only way to gain 100 percent certainty is by way of manual rework. The real issue here is that you need to redo the work for 100percent of all the records, not just for the incorrect 20percent. This effectively renders the AI obsolete.
However, in certain steps of the analytics value chain AI performs more efficiently and quickly than even the smartest data scientists. AI is particularly helpful when large amounts of data or information are involved. On the other hand, the human mind is still crucial when it comes to the ability to spot and react to new patterns (as opposed to known patterns);understand the broader business context; differentiate between correlations and root-cause relationships; and tell machines what to do. These traits are key ingredients for creating insights or knowledge and will continue to be dominated by humans, at least in the foreseeable future.
How can we make this mind+machine collaboration work profitably for many, vastly different and dynamic analytics applications? The answer is by eliminating clutter. End users do not have the time to review lengthy data sets or reports filled with information. They want concise insights to inform their decisions. Useful AI-based algorithms can plough through large data sets, remove the unnecessary clutter, and provide results by relevance. This is where the machine works best and accomplishes the task that humans would not be able to do at all (or only very slowly). A human analyst reviews the results offered by the machine and make a final decision on whether they are relevant or not. This judgment is end-user specific and adds context to the result – something that is still out of reach for even the most intelligent algorithms.
If each decision the human analyst makes gets incorporated into the machine through machine-learning techniques, the algorithm may get better at deciding the level of relevance. Only the results that have been validated by the analysts will then be passed on to the end user. Helpful as it may be, AI currently contributes to only a part of the value chain. The vast majority of AI applications still need a mind element to create truly useful insight. On top of this, most analytics solutions include many other machine elements, such as tools for workflow, productivity, dissemination, and knowledge management. AI is usually just the icing on the cake. Rather than being the answer to every analytics question, AI can be a very powerful tool when used appropriately.