
How 'Noise' can help Businesses Close the Last Mile in Artificial Intelligence

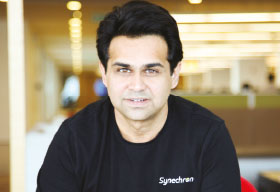
And, AI has the power to address these challenges and predict outcomes like never before, but it will require deep domain expertise to cover this “last mile.”
Third Generation Artificial Intelligence
The 21st Century is presently transitioning into the Era of Deep Learning, where leaders like Google are using deep learning algorithms in neural networks find out impacting member from data and use that data to predict a model. These systems flag data to neural networks and work on a concept called ‘auto encoding’ which tries to find a correlation between different data points. Most are “noise” data points. The technique uses recurrent neural networks (RNN) to allow the system to auto-correlate historical data and live feeds. For example, if a company took historical data from the top 10 countries by Gross Domestic Product (GDP) with employment ratios, inflation data, gold prices, and stock exchange data and put it all into a historical system, along with live feeds, the neural network would auto-correlate the data.
As with any system, the analysis will only be as good as the data; how ever, the challenge with deep learning AI systems is that in most cases, the historical data was not collected with AI systems in mind. Instead, that data is routinely altered for usability within business systems. Deep learning algorithms, however, need data as close as possible to the original – flaws and all – for optimal predictive modeling. And importantly, they cannot discount the “noise,” which given the right business domain knowledge could actually be factors that have an unfor seen impact on the data.
What is 'noise' data?
In engineering, raw, pure, 'analogue,'data includes three core factors: real-time (velocity), noise (variety)and full information (volume). In AI,"noise" would then be any data (whether intended or not) that gives environmental context. To take a simple example, if you see a photo of a car and a person you cannot tell if the car will hit the person, but if you then find out it is a video
and when unpaused, the car is slowly moving toward the person, you can predict the individual is likely to be hit by the car. This is important contextual information or 'noise.'
In the financial services industry, Machine Learning to be used to find a correlation between spending patterns and the banking needs of a person - in an area such as forex. Generally, systems look at spending data and locations and 'noise' data like cash spend are not factored. However, by using the customers' historical spend data you can at maximum predict what his future spend will be.
The challenge is, this type of data is often unintentionally filtered out when being moved from paper to digital. AI results will be the most accurate if the source data includes the original "noise." There fore, businesses need to understanding their data fidelity – where did the data come from and how much has it changed in order to determine how much value to give to correlations and predictions. This may not be acceptable for business systems but is essential for the AI model, where the measure of data fidelity will directly impact the accuracy of the predictive model. This will need to be applied across all data fed into the deep learning model with data variance.
Understanding data fidelity and then the meaning of predictions once they are made requires deep domain expertise. It is, therefore, this combination of techno-functional expertise that will allow businesses to reach the full potential of AI technologies. This is what I like to call the 'last mile of AI adoption'. Clearly, companies like IBM, Google, Intel, Facebook, and many others have made tremendous progress in the field of AI technology innovation, but the last mile is applying this technology to specific business verticals with an applied approach. For the data to be meaningful, the algorithms to be selected and tuned, and the operations set up, the experts also will need to understand front-, middle-, and back-office business operations.
While there is tremendous value in having a PHD as part of the team, domain experts will be critical when it comes to setting up data lakes to inform the algorithms and defining the business problem. With a techno-functional approach drawing on domain expertise in an industry like financial services, businesses can reach beyond human intelligence to solve new challenges, automate processes, meet compliance objectives, and accelerate their business growth. These changes might range from common use cases like anti-money laundering to much more complex, specialist business cases like coming up for a predictable price around illiquid OTC products. Yes, this will impact today’s jobs, so companies will also need to think about how they re-skill their workforce to elevate their employees to take on new roles by training them for the jobs of the future.
About Faisal Husain
After having identified huge gaps in the technology consulting and outsourcing marketplace, Faisal co-founded Synechron in 2001, to provide customized, client centric solutions provided by subject matter experts with a deep domain understanding. Synechron,a $450 million firm based in New York, is today one of the fastest growing digital, business consulting & technology services providers. Grown on its own back since inception in 2001, Synechron has consistently been on a steep growth trajectory - north of 25 percent. With 7,500+ professionals operating in 18 countries across the world, it has presence across USA, Canada, UK, France, The Netherlands, Switzerland, Luxembourg, Serbia, Hungary, Germany, Italy, UAE, Singapore, Hong Kong, Philippines, Japan, Australia and Development Centers in India.
In the financial services industry, Machine Learning to be used to find a correlation between spending patterns and the banking needs of a person - in an area such as forex. Generally, systems look at spending data and locations and 'noise' data like cash spend are not factored. However, by using the customers' historical spend data you can at maximum predict what his future spend will be.
Businesses need to understand their data fidelity – where did the data come from, and how much has it changed, in order to determine how much value to give to correlations and predictions
The challenge is, this type of data is often unintentionally filtered out when being moved from paper to digital. AI results will be the most accurate if the source data includes the original "noise." There fore, businesses need to understanding their data fidelity – where did the data come from and how much has it changed in order to determine how much value to give to correlations and predictions. This may not be acceptable for business systems but is essential for the AI model, where the measure of data fidelity will directly impact the accuracy of the predictive model. This will need to be applied across all data fed into the deep learning model with data variance.
Understanding data fidelity and then the meaning of predictions once they are made requires deep domain expertise. It is, therefore, this combination of techno-functional expertise that will allow businesses to reach the full potential of AI technologies. This is what I like to call the 'last mile of AI adoption'. Clearly, companies like IBM, Google, Intel, Facebook, and many others have made tremendous progress in the field of AI technology innovation, but the last mile is applying this technology to specific business verticals with an applied approach. For the data to be meaningful, the algorithms to be selected and tuned, and the operations set up, the experts also will need to understand front-, middle-, and back-office business operations.
While there is tremendous value in having a PHD as part of the team, domain experts will be critical when it comes to setting up data lakes to inform the algorithms and defining the business problem. With a techno-functional approach drawing on domain expertise in an industry like financial services, businesses can reach beyond human intelligence to solve new challenges, automate processes, meet compliance objectives, and accelerate their business growth. These changes might range from common use cases like anti-money laundering to much more complex, specialist business cases like coming up for a predictable price around illiquid OTC products. Yes, this will impact today’s jobs, so companies will also need to think about how they re-skill their workforce to elevate their employees to take on new roles by training them for the jobs of the future.
About Faisal Husain
After having identified huge gaps in the technology consulting and outsourcing marketplace, Faisal co-founded Synechron in 2001, to provide customized, client centric solutions provided by subject matter experts with a deep domain understanding. Synechron,a $450 million firm based in New York, is today one of the fastest growing digital, business consulting & technology services providers. Grown on its own back since inception in 2001, Synechron has consistently been on a steep growth trajectory - north of 25 percent. With 7,500+ professionals operating in 18 countries across the world, it has presence across USA, Canada, UK, France, The Netherlands, Switzerland, Luxembourg, Serbia, Hungary, Germany, Italy, UAE, Singapore, Hong Kong, Philippines, Japan, Australia and Development Centers in India.